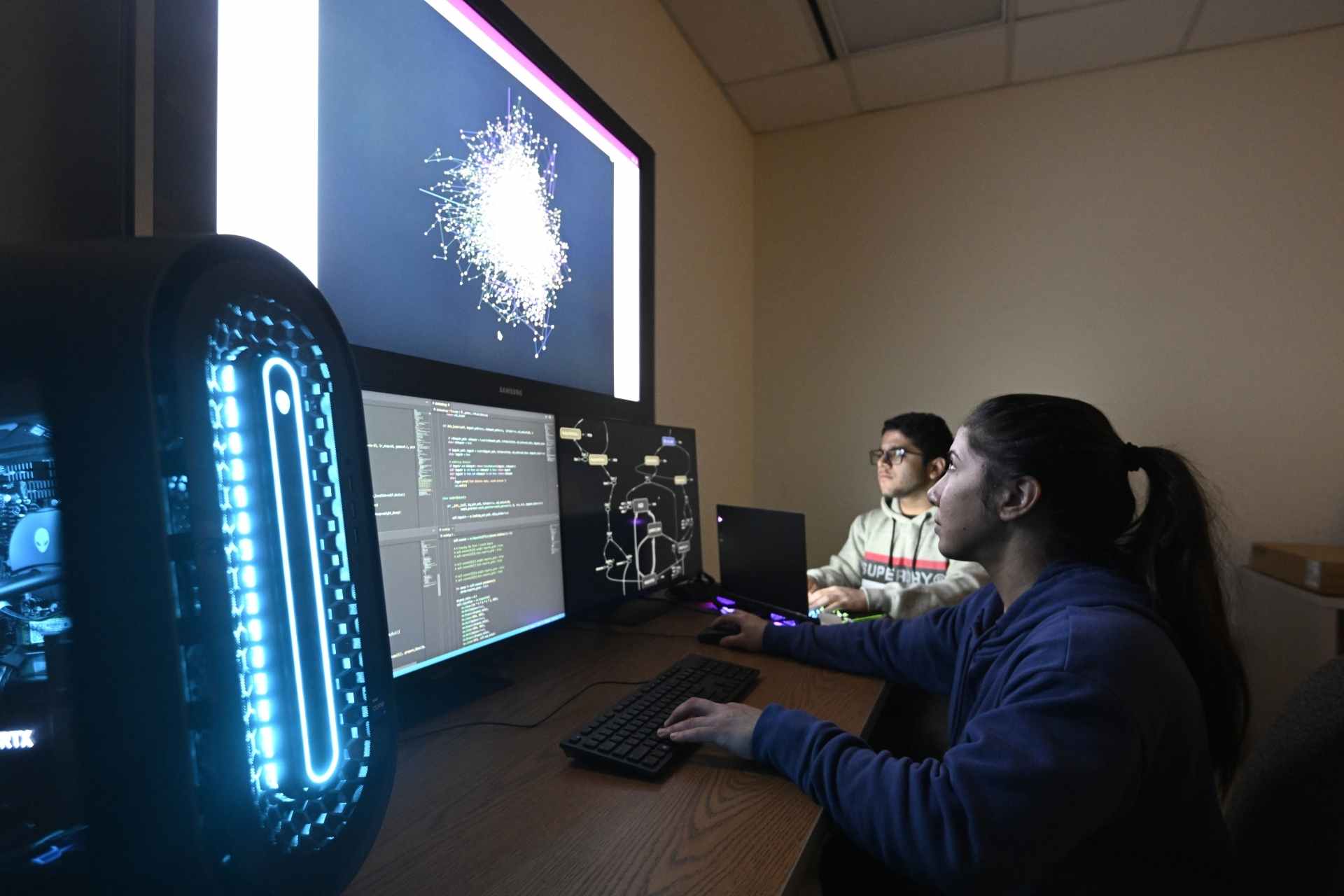
Electric fish and robots may hold the key to achieving “autonomous lifelong machine learning,” based on research conducted at West Virginia University with the aid of a $2 million National Science Foundation grant.
Computer scientist and engineer Gianfranco Doretto is leading a team of researchers in a four-year study of unsupervised continual machine learning, applying the results of experiments on electric fish to the development of software that will allow a robot to learn to navigate different terrains unaided.
Doretto, an associate professor at the Benjamin M. Statler College of Engineering and Mineral Resources, is seeking inspiration not in human brains, but in the neuroscience behind how electric fish process stimuli and learn from them.
Doretto knows that for artificial intelligence to operate independently in the real world, it needs to be capable of flexible, resilient, ongoing learning.
“A living creature exploring the world experiences the world’s dynamics and uncertainty,” Doretto said. “To survive, it constantly adapts. This is lifelong learning that living beings do without any coaching or supervision.
“We want to build agents — for instance, robots — that, like living beings, possess lifelong learning abilities which allow them to continuously adapt their behavior based on how they sense the world as they explore and interact with it.”
Electric fish enter the picture because they “generate an electric field around their bodies to navigate, similar to bats’ sonar,” he explained. The field generated by the electric fish involved in this study isn’t strong enough to shock anything, but it allows the fish to sense objects and movement: rocks, weeds, prey, predators. Neuroscientists love electric fish because those fields make visible some aspects of neurosensory processing.
For example, electric fish studies have shown how, in order to learn about the world, a fish “filters out self-generated sensory inputs,” Doretto said — erasing from consciousness signals created by its own body or movements. Canceling that input isn’t something current machine learning can do.
Electric fish have also shown they can generalize from specific stimuli, applying things learned in one environment or situation to another. Machines need that skill, too.
By surrounding the fish with other fish to trigger changes in background noise, or by altering water conductance through the simulation of a rainy season, the researchers will see how the fish’s neural networks adapt, then enable similar processes for machine learning.
Presently, machine learning happens in controlled, supervised conditions, with software programs passively absorbing datasets in offline mode before they go online to apply what they’ve learned. That approach makes it possible for current AI “to perform a large variety of tasks, from translating between languages, to providing automated services as an internet bot, making diagnoses from medical records or driving autonomous cars,” Doretto said.
But current AI isn’t good at doing something as seemingly trivial as switching from walking on a hard floor to walking on dry sand.
Doretto said the current state of machine learning requires human intervention, where a robot would be taken out of the field and retrained to walk on sand, retraining that can cost time and money.
His team will enable a robot to learn autonomously and in real time how to change its way of walking so it will effectively navigate on sand without having done it before.
Fundamental to that achievement will be endowing the AI with the ability to learn and forget at different speeds, just as “living beings learn and forget certain things very quickly and others more slowly,” Doretto said.
A significant advantage of learning and forgetting on flexible timescales is the avoidance of “catastrophic forgetting,” a major machine learning challenge that occurs when an AI forgets what it learned from previous data after being trained on new data.
Doretto pointed out that “most AI technologies, when deployed in real applications, process data that are statistically different from the data used in training. This causes the AI to become less accurate or even dangerous to rely on.”
By enabling continually adaptive machine learning, Doretto’s research can ensure an AI’s predictions become more accurate over time.
The element of surprise is crucial to making that kind of learning possible.
“Neuroscientists seem to agree that primate brains support interaction with the environment by continuously monitoring for sensory input that is novel or unpredictable,” Doretto said.
“This ‘surprising’ input is responsible for significant neural activity, which in turn drives neural ‘plasticity’ — the ability of neural networks to continuously reshape themselves.”
As Doretto’s robot explores the world, it will “sense what is surprising,” reshaping its neural networks as it learns and forgets more quickly or slowly.
As soon as the software is ready, the AI will get its legs and the artificial neural network will be connected with a multisensory legged robotic platform.
Researchers will put this robot through its paces, having it learn to walk first on a solid surface, then on sandy terrain. It will master motions by itself, using an ongoing flow of real-time sensory feedback from multiple sources like the rotation of its joints or the bending of its leg.
Core collaborators at WVU are Nicholas Szczecinski, assistant professor of mechanical engineering, Donald Adjeroh, professor and associate chair for faculty development and graduate coordinator for computer science, and Nasser Nasrabadi, professor of computer science and electrical engineering, all of Statler College; Gary Marsat, associate professor of neuroscience at Eberly College of Arts and Sciences; and Valarie Blake, professor and associate dean for faculty development and research at the College of Law.
Field robotics applications for the machine learning software could include space exploration; agricultural tilling, planting and harvesting; underground mining and mineral extraction; construction and fabrication tasks; and search and rescue operations.
As Doretto said, “Our research could provide a transformative contribution anywhere robots need to perform laborious or dangerous tasks for humans where the environment is unstructured and unexplored.”
"fish" - Google News
November 07, 2022 at 10:33AM
https://ift.tt/bd7rz1I
Neuroscience of electric fish gives jolt to advances in machine learning at WVU - My Buckhannon
"fish" - Google News
https://ift.tt/DdTfpcx
https://ift.tt/SmgAFuh
Bagikan Berita Ini
0 Response to "Neuroscience of electric fish gives jolt to advances in machine learning at WVU - My Buckhannon"
Post a Comment